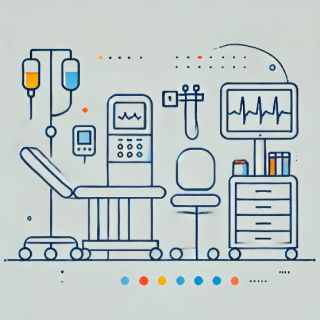
Supply: DALL-E / OpenAI
The yr 2025 might effectively mark a pivotal second within the evolution of artificial intelligence (AI) in medication. A new prepress study evaluating OpenAI’s GPT-4 and o1-preview mannequin demonstrates that AI isn’t solely reaching spectacular feats in medical reasoning however is doing so with out supplemental coaching on domain-specific knowledge. This achievement represents a vital leap in what general-purpose giant language fashions (LLMs) can accomplish, fueled by improvements in reasoning frameworks comparable to chain-of-thought (CoT) processing.
The findings are each promising and provocative. On one hand, the o1-preview mannequin excels in duties requiring advanced diagnostic and management reasoning, rivaling human clinicians. On the opposite, it reveals essential gaps in probabilistic reasoning and triage analysis, areas the place human experience stays paramount. This duality raises necessary questions on how AI will combine into medical workflows and redefine the function of clinicians.
There’s a lot to unpack right here, and I recommend studying the research fastidiously as I am solely referring to among the key factors, notably the outcomes with the o1-preview mannequin.
A Story of Strengths and Weaknesses
The research evaluated the o1-preview mannequin throughout 5 experiments, together with differential analysis technology, diagnostic reasoning, triage differential analysis, probabilistic reasoning, and administration reasoning. The outcomes had been adjudicated by doctor consultants utilizing validated psychometrics, offering a benchmark for comparability towards human controls.
Strengths:
-
Differential analysis technology: The o1-preview mannequin achieved an 88 % accuracy fee, far surpassing the 35 % accuracy demonstrated by human clinicians in the identical process. Its output was persistently rated as extra complete and exact, notably in uncommon and sophisticated diagnostic situations, the place the mannequin’s CoT reasoning allowed it to determine situations usually ignored by clinicians.
-
Diagnostic and administration reasoning: The o1-preview mannequin displayed vital developments in diagnostic and administration duties. In 84 % of instances, the mannequin’s reasoning was rated as on par with or exceeding that of human consultants, who achieved comparable accuracy in solely 64 % of instances. Physicians praised the mannequin’s structured and logical strategy, which mirrored the stepwise essential considering employed by clinicians and synthesized knowledge from various medical inputs to supply actionable suggestions.
Limitations:
-
Probabilistic reasoning: The mannequin struggled with duties requiring nuanced probabilistic reasoning—a cornerstone of medical decision-making. Whereas the o1-preview mannequin’s efficiency was according to prior LLMs, human clinicians continued to excel on this space, demonstrating larger adaptability in assigning likelihoods to competing diagnoses and dynamically balancing dangers in unsure conditions.
-
Triage differential analysis: No enhancements had been noticed in triage duties that require prioritizing instances by severity. Whereas human clinicians achieved a 70 % accuracy fee in these high-pressure, dynamic situations, the mannequin’s logical however inflexible outputs fell quick, missing the adaptive nuance required for real-time decision-making in emergency or essential care settings.
The Position of Chain-of-Thought Reasoning
A standout function of the o1-preview mannequin is its reliance on CoT reasoning, a framework that permits the AI to generate intermediate steps in its reasoning course of earlier than arriving at a remaining reply. This course of permits the mannequin to clarify its thought course of, making its outputs extra clear and simpler for clinicians to interpret.
By breaking down advanced issues into smaller steps, CoT reasoning reduces the chance of logical errors, notably in duties requiring essential considering. Furthermore, this strategy mimics the way in which clinicians handle diagnostic challenges—systematically contemplating signs, take a look at outcomes, and medical historical past to type conclusions. The usage of CoT reasoning could also be an necessary issue within the mannequin’s success with diagnostic and administration reasoning, even because it struggles with the extra dynamic features of medical observe, comparable to triage.
The Exceptional Absence of Supplemental Medical Coaching
One other hanging side of the o1-preview mannequin is that it was not skilled on supplemental medical knowledge. In contrast to earlier AI programs fine-tuned on medical knowledge units, o1-preview achieved its efficiency utilizing general-purpose coaching. This accomplishment means that broad, basic coaching knowledge mixed with superior reasoning frameworks can rival domain-specific coaching, decreasing the necessity for expensive and time-intensive fine-tuning processes.
The absence of supplemental coaching additionally eliminates issues about affected person privateness, biased knowledge units, and overfitting to particular situations. Nevertheless, it means the mannequin’s efficiency is restricted to patterns current in its basic coaching knowledge, leaving gaps in areas requiring contextual nuance. This highlights each the promise and the present limitations of generalist AI programs in specialised domains like healthcare.
A Wake-Up Name for Clinicians
The o1-preview mannequin’s efficiency highlights each the promise and the constraints of LLMs in medication. For clinicians, this research serves as a wake-up name: AI is now not a futuristic idea—it’s right here, and it’s redefining what is feasible in affected person care.
-
AI as a accomplice: Fashions like o1-preview usually are not changing clinicians however augmenting their capabilities. They excel at duties like differential analysis technology and administration planning, liberating up clinicians to deal with affected person interplay and decision-making.
-
Closing the gaps: Whereas o1-preview shines in structured reasoning duties, its struggles with probabilistic reasoning and triage emphasize the irreplaceable worth of human experience. These gaps level to alternatives for future AI growth.
-
The necessity for new benchmarks: Present analysis strategies, comparable to multiple-choice query benchmarks, fail to seize the complexity of real-world medical situations. Strong, scalable benchmarks and medical trials are important to grasp AI’s true potential in healthcare.
Digital Well being and “One other” Inflection Level?
The o1-preview mannequin might characterize a turning level within the integration of AI into medication. And as we have heard this declare many occasions, its means to carry out superhuman reasoning duties with out supplemental medical coaching is necessary—as an achievement and a problem. As AI continues to evolve, clinicians should adapt to this new actuality, embracing AI as a cognitive accomplice whereas sustaining the human experience that defines the artwork of drugs.
2025 is not only a wake-up name—it might be the start of a new period. The query is now not whether or not AI will remodel medication, however how clinicians and AI will work collectively to form the way forward for healthcare.